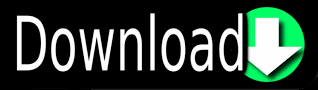
str = unique_strings (index) % Calculate the information, % given probability of the current str.Featured on Meta Planned maintenance scheduled for Thursday, September 2 at 12:00am UTC… In first case, b is an array of 5 symbols. Designed to be trained and applied via convolution over an entire image. Here's an example: hair= entropyF(class,hair) ans = 0. First, notice that I specified a neigborhood using a disc-shaped structuring element (strel) of radius 1. m This program generates a pseudorandom sequence of a given length from a given alphabet with prescribed symbol frequencies.
The algorithms are based on an adaptive importance sampling method called the improved cross-entropy method developed in Chan and Kroese (2012). For instance, CCE uses CE and SE functions.
#WHAT IS CROSS ENTROPY CODE#
The evidence I find suggests that MATLAB Compiler did not exist until 1995, making it unlikely that the original code was MATLAB and compiled by MATLAB Compiler.
Entropy is a lower bound on the average number of bits needed to represent the symbols (the data compression limit). The classical Maximum Entropy (ME) problem consists of determining a probability distribution function (pdf) from a finite set of expectations of known functions. The solution depends on Lagrange multipliers which are determined by solving the set of nonlinear equations formed by the data constraints and the normalization constraint. Aimal Rehman BTE-SP12-038 Department of Electrical Engineering, CIIT Lahore. Considers Huffman coding and arithmetic coding. function apen = Fuzzy_entropy_bbd ( n, r, a) %% Code for computing approximate entropy for a time series: Approximate. PSNR and MSE parameters can be used for the same which I have implemented for looking for matlab code on following performance measurement parameters Entropy Correlation = RXY ( α, β )= ∑ i ∑ j X(i,y) Y(i- α ,j- β ) I am trying to understand the concept of Shannon's entropy and deciding the codelength. Added checks to ensure input MATLAB types are doubles. Compute the cross-entropy loss between the predictions and the targets. Sparse cross-entropy can be used in keras for multi-class classification by using….% fluctuations in a time series. Sparse cross-entropy addresses this by performing the same cross-entropy calculation of error, without requiring that the target variable be one-hot encoded prior to training. This can mean that the target element of each training example may require a one-hot encoded vector with thousands of zero values, requiring significant memory. It is frustrating when using cross-entropy with classification problems with a large number of labels like the 1000 classes. output like is a valid one if you are using binary-cross-entropy. pile(loss='categorical_crossentropy', optimizer=opt, metrics=)ĭifferent between binary cross-entropy and categorical cross-entropyīinary cross-entropy is for binary classification and categorical cross-entropy is for multi-class classification, but both work for binary classification, for categorical cross-entropy you need to change data to categorical( one-hot encoding).Ĭategorical cross-entropy is based on the assumption that only 1 class is correct out of all possible ones (the target should be if the 5 class) while binary-cross-entropy works on each individual output separately implying that each case can belong to multiple classes( Multi-label) for instance if predicting music critic contains labels like Happy, Hopeful, Laidback, Relaxing, etc. Model.add(Dense(10, activation='softmax'))
The example of Binary cross-entropy loss for binary classification problems is listed below. The output layer needs to configure with a single node and a “ sigmoid” activation in order to predict the probability for class 1. Output size is the number of scalar values in the model output. It calculates the loss of an example by computing the following average: The score is minimized and a perfect value is 0. It will calculate a difference between the actual and predicted probability distributions for predicting class 1. It is intended to use with binary classification where the target value is 0 or 1. In this tutorial, you will discover three cross-entropy loss functions and “how to choose a loss function for your deep learning model”. Further, the configuration of the output layer must also be appropriate for the chosen loss function. The choice of loss function must specific to the problem, such as binary, multi-class, or multi-label classification. This requires the choice of an error function or loss function, that can be used to estimate the loss of the model so that the weights can be updated to reduce the loss on the next evaluation. In the Deep Learning error of the current state must be estimated repeatedly.